9 Strategies for Handling Large Volumes of Data
ITInsights.io

9 Strategies for Handling Large Volumes of Data
Navigating the complexities of data management requires more than just powerful tools; it demands wisdom from those at the forefront of the industry. This article delves into proven strategies for handling large volumes of data, enriched with insights from seasoned experts. Discover how to transform data challenges into opportunities by leveraging innovative solutions and expert advice.
- Leverage Cloud-Based Solutions
- Focus on Automation and AI
- Implement Effective Data Warehousing
- Utilize Cloud-Based Data Warehousing Solutions
- Adopt Serverless Architecture for Data Processing
- Organize Data with Predictive Analytics
- Use Scalable Distributed Systems
- Implement Source Deduplication and CDP
- Harness Machine Learning for Predictive Analytics
Leverage Cloud-Based Solutions
At NetSharx Technology Partners, we handle large volumes of data through our expertise in cloud-based solutions, particularly using scalable SDWAN and SASE networks. This allows us to support extensive data flows efficiently while maintaining robust security measures across distributed environments. With access to over 350 cloud providers, we can tailor data management solutions that are unique to each organization's needs.
For instance, when working with a global manufacturing company, we helped improve their Azure application performance, achieving consistent sub-100 ms latency across their network by prioritizing interconnection strategies. This not only minimized network latency from 13 ms to 3 ms but also enabled us to automate service delivery in under four hours. This approach is a game-changer for businesses needing real-time data processing and improved user experiences.
By leveraging our agnostic approach to cloud solutions, we help organizations quickly migrate and manage data workflows seamlessly, cutting down time and complexity. The keys are custom solutions, and robust networks, ensuring that data flows optimally without the burden of conventional infrastructure constraints.At NetSharx Technology Partners, we handle large volumes of data by leveraging cloud-based technologies and strategic data consolidation. One effective strategy is implementing a scalable SD-WAN solution that improves our network's flexibility and security while optimizing data flow. This technology enables us to swiftly adapt to growing data demands and ensure efficient data management across various platforms.
A specific example of our data management strategy is our collaboration with Equinix to improve latency and network performance. By using Platform Equinix and Microsoft Azure, we reduced latency by up to 59% in some locations, which significantly improved the performance of our cloud services. This approach ensures that we can handle high data volumes with improved efficiency and user experience.
We also emphasize the importance of real-time data processing through cloud contact center platforms with built-in KPI tracking. These platforms allow us to monitor and quickly respond to data-driven insights, ensuring seamless end-to-end data management. This strategic alignment helps us manage big data while maintaining focus on enhancing overall service delivery.
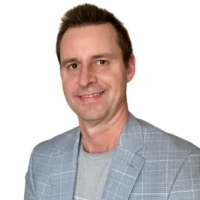
Focus on Automation and AI
At SuperDupr, we've honed our approach to managing large volumes of data by focusing on automation and AI integration. One strategy we advocate is leveraging machine learning algorithms to analyze and predict data patterns, helping us optimize processes for our clients. This not only streamlines operations but also offers valuable insights, thus enhancing efficiency.
A concrete example of our data management approach is seen with a client, Goodnight Law, where we designed a customized email system integrated with auto-follow up features. By automating this process, we managed high volumes of communication data effectively, increasing conversion rates and client engagement without overwhelming our systems.
Our unique process methodology focuses on enhancing client satisfaction by ensuring that data-driven decisions are implemented seamlessly. Through partnerships with leading tech providers, we integrate cutting-edge technologies custom to handle our clients' specific data needs, allowing them to scale without data-related bottlenecks. In managing large volumes of data, I focus on process automation and optimization using AI. At SuperDupr, we've refined our methodology to automate and scale digital processes effectively. For instance, we've helped clients like Goodnight Law revamp their systems, enhancing data handling capabilities and operational efficiency by integrating automated email follow-ups and data-driven strategies. This approach has drastically reduced manual work and improved accuracy, leading to higher client satisfaction.
One effective strategy is automating lead generation and client tracking. By employing AI-driven solutions, we're able to sift through vast client data swiftly, prioritizing potential leads and optimizing conversion processes. This reduces the human resource requirement for data processing and improves our ability to deliver quick and efficient client service, a crucial aspect for our growth and client success at SuperDupr.
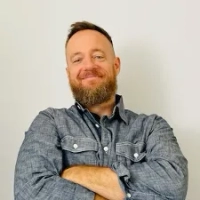
Implement Effective Data Warehousing
When dealing with large volumes of data, I focus on effective data warehousing and integration strategies. In my role at Profit Leap, I've implemented centralized data warehouses that store information from diverse sources. This setup ensures that all data is organized and accessible, allowing for efficient analysis.
Additionally, using AI-powered solutions like Huxley has been instrumental. By integrating this AI business advisor, we've streamlined the process of extracting actionable insights from our data, enabling businesses to make informed decisions quickly. This approach not only increases efficiency but also improves the accuracy of our data-driven strategies.
A specific example involves working with a diagnostic imaging firm, where we used Hadoop clusters to manage and process extensive medical imaging data, significantly reducing processing time. This resulted in operational improvements and allowed us to deliver timely insights, which were crucial for strategic decision-making in healthcare. One of the strategies I leverage in managing large volumes of data is through the use of an AI business advisor, specifically, our own tool called Huxley. Huxley helps streamline data from multiple sources, providing real-time, actionable insights through customized dashboards. This allows small businesses to make data-driven decisions efficiently and effectively without needing a deep technical background.
For instance, while working with a small law firm, we implemented Huxley to consolidate client and financial data. It resulted in a 50% year-over-year revenue increase by enabling the firm to identify underused services and optimize resource allocation. The tool's ability to predict trends based on historical data gave the firm a competitive edge.
I often combine these AI tools with a data-driven strategic framework I developed, called the 8 Gears of Success, which emphasizes diagnosing business challenges and tailoring solutions to ensure data accuracy and reliability. This approach doesn't just handle data but transforms it into a catalyst for growth and innovation.
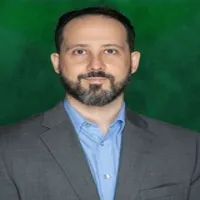
Utilize Cloud-Based Data Warehousing Solutions
Handling large volumes of data requires a scalable, efficient strategy, and one effective approach is leveraging cloud-based data warehousing solutions like Google BigQuery or Snowflake. These platforms enable real-time processing, seamless integration, and advanced analytics without infrastructure limitations. For example, using BigQuery, I streamlined marketing campaign data analysis by running complex queries quickly, optimizing ad spend, and improving targeting. The key to managing big data is combining automation, cloud scalability, and structured data organization for actionable insights and efficiency.
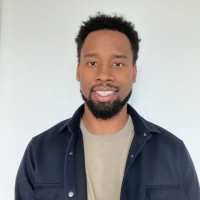
Adopt Serverless Architecture for Data Processing
Handling large volumes of data effectively requires a mix of efficient technologies and structured processes to ensure everything is stored, processed, and analyzed properly. One strategy we use to manage big data is leveraging serverless architecture combined with AWS Lambda for processing.
By using serverless computing, we're able to run backend functions in response to data events (like incoming data streams) without having to manage servers. This setup lets us automatically scale our data processing infrastructure based on how much data is coming in, which reduces both cost and complexity. For example, when large datasets are uploaded, Lambda functions are triggered to process and clean the data, making it ready for analysis or other operations, all without needing manual intervention or server maintenance.
This approach has allowed us to reduce operational overhead, optimize resource usage, and speed up data processing. As a result, our teams can focus more on extracting insights and less on managing infrastructure. Plus, it gives us the flexibility to handle fluctuating data volumes with minimal manual work-something that's key in a fast-growing business with dynamic data needs.
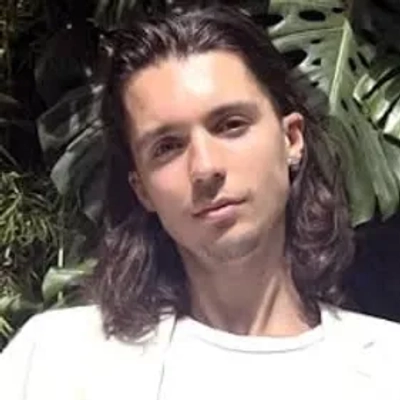
Organize Data with Predictive Analytics
Handling large volumes of data starts with organization. At Tech Advisors, we set up structured and unstructured data storage solutions tailored to each business. A well-maintained data warehouse helps businesses access structured data quickly, while a data lake allows for the storage of raw, unstructured information that can be processed later. Many businesses struggle with data overload, but sorting data from the start--labeling what's important and filtering out unnecessary noise--makes analysis faster and more accurate.
One effective strategy we use is predictive analytics. Many businesses collect years of data but don't know how to use it. We help them analyze past trends and behaviors to anticipate future needs. For example, a client in financial services needed a way to detect fraudulent transactions before they happened. By using predictive analytics, we helped them identify suspicious patterns, reducing fraud incidents significantly. This approach also works well in cybersecurity, where detecting unusual network activity early can prevent data breaches.
Big data management isn't just about storage and analysis; security is just as important. We've seen companies suffer because they didn't take the right precautions. One key lesson is restricting access--only the right people should have sensitive information. Encryption and regular audits also play a major role. At Tech Advisors, we always remind clients that data security isn't a one-time fix; it's an ongoing effort that protects both businesses and their customers.
Use Scalable Distributed Systems
At FusionAuth, we handle large volumes of user data by leveraging scalable, distributed systems. One technology I rely on is sharded databases, which distribute data across multiple servers. This setup reduces the load on individual databases, ensuring that as user numbers grow, performance remains stable.
Another key strategy is caching user session data using technologies like Redis. By storing session data in a dedicated cache, we decrease the load on our databases, improving response times for user authentication requests. Caching allows us to maintain speed and efficiency even as we manage thousands of concurrent login attempts.
A practical example of this in action was when we worked with our clients to scale authentication systems to handle over 7,000 concurrent logins. We optimized database queries, used read replicas, and integrated caching to manage the load effectively. This approach significantly improved the performance and reliability of their systems.
At FusionAuth, managing large volumes of data is crucial for providing seamless authentication services. One technology that has proven effective for us is caching. We use Redis for caching user sessions, which significantly reduces our primary database's load, ensuring that login and authentication processes remain fast and reliable. By distributing sessions across a caching layer, we're able to handle high traffic and prevent bottlenecks in real time.
For data distribution and storage, utilizing sharding and read replicas has been instrumental. For instance, when AdCellerant migrated over 100,000 user attributes to FusionAuth, these techniques ensured the process was seamless and scalable. Sharding allows us to segment data intelligently, while read replicas help distribute the load, ensuring that our database architecture can efficiently handle increased data and user queries.
Digitally signed JWT tokens also aid in handling large data volumes without frequent database checks. By facilitating decentralized token verification, we're able to scale horizontally, allowing different parts of the system to independently verify tokens. This reduces the dependency on central authorization servers and improves latency, ensuring efficient user verification without the need for constant database access.
Implement Source Deduplication and CDP
Handling large volumes of data is all about leveraging the right technology to streamline processes and optimize efficiency. At ETTE, we specialize in cloud-based data management solutions. The flexibility of cloud computing allows us to scale resources as needed, making it easier to store and process big data without hefty upfront investments.
One effective strategy I use is source deduplication, which reduces storage costs and ensures efficient backup processes. It's comparable to packing efficiently for a trip, where I only keep what's necessary, eliminating redundancy. This approach facilitates faster backups and retrievals, improving overall data management performance.
We also implement global backup consolidation into a single data lake. This enables a unified data repository that improves accessibility and recovery speed. By consolidating backups, we simplify compliance and eDiscovery processes, ensuring robust and efficient data protection strategies that are vital in today's digital landscape.Handling large volumes of data is something I'm deeply involved in through my work at ETTE, where we specialize in IT solutions for nonprofits and small businesses. One strategy I've found effective is Continuous Data Protection (CDP). This technology backs up every data change in real-time, ensuring that no significant activity is ever lost. We implemented CDP for a nonprofit client handling sensitive and dynamic data, allowing them seamless operations without worrying about data losses during critical fundraising events.
Another approach is consolidating global backups into a single data lake. By bringing various data sources together into one searchable repository, we improve backup and recovery efficiency. This strategy was applied for a client with multiple office locations, simplifying their data management and boosting their operational efficiency. This consolidation ensures that disaster recovery is streamlined, minimizing downtime and allowing for quick restoration processes.
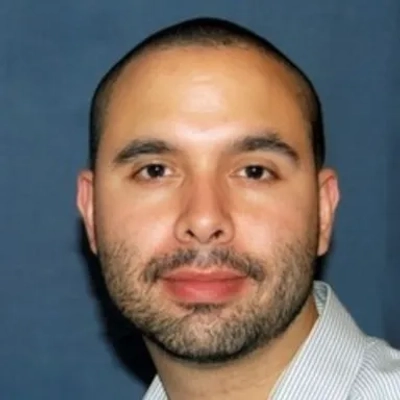
Harness Machine Learning for Predictive Analytics
As someone who has transformed operations for enterprises with massive datasets, I've harnessed the power of advanced analytics and big data to drive results effectively. A strategy that stands out is leveraging machine learning for predictive analytics. By implementing data ingestion techniques, we could handle and analyze unstructured data efficiently. This approach enabled us to predict demand shifts and optimize operations in real-time.
For example, by working closely with large tech enterprises, we integrated multi-source data analytics to streamline operations, leading to improved decision-making and predictive power. This process allowed for a 16% improvement in delivery times, providing a strategic advantage by reducing costs and boosting customer satisfaction.
In UpfrontOps, data handling is crucial for maintaining our explosive growth, especially as we work with 4,500+ global B2B technology brands. By creating a centralized team for data management, we improved data quality and operational efficiency, which is foundational to driving significant improvements in our analytics capabilities and competitive positioning. Handling large volumes of data efficiently is a critical aspect of my work at UpfrontOps. One strategy I use is implementing enterprise-wide analytics solutions, which involves integrating machine learning and real-time data analytics. By doing this, we not only streamline our data processes but also significantly reduce errors and manual workloads.
An example from my experience is our collaboration with AT&T and AWS to deploy a cloud-based solution that improves data ingestion and processing capabilities. This system allowed us to handle vast datasets seamlessly, resulting in a 16% improvement in operational efficiencies and a dramatic increase in service reliability.
We also focus on end-to-end visibility for supply chains, leveraging AI to integrate data from various sources. This comprehensive approach was instrumental in achieving a 40% improvement in delivery accuracy for a logistics client, providing them with a considerable competitive advantage in their market.
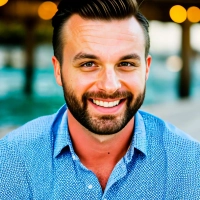